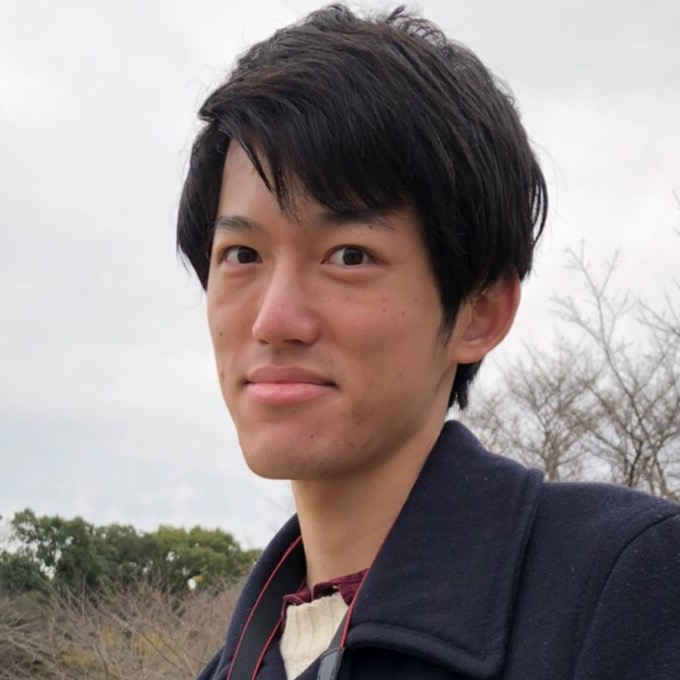
-
Period12th(Term; from Oct. 2021)
グローバル型 -
Research InterestsStatistical Machine Learning
-
Research TopicVerifiable Machine Learning
-
Host DepartmentGraduate School of Informatics
-
Previous AffiliationGraduate School of Information Science and Technology, The University of Tokyo
As modern computational powers and resources enable us to collect a vast amount of data, data-driven knowledge discovery has become more and more important in science and decision making. Statistical machine learning is one of the key components to form the basis of such inference, by implementing statistics-based inductive inference on modern computers.
While machine learning has been successful in various domains such as game AI and autonomous driving, it is challenging to guarantee the performance of inductive inference, which hinders machine learning from real-world applications in risk-sensitive domains.
I have been working on establishing a theoretical framework to verify whether our inference model can achieve the expected performance or not under the situations where the collected data is largely skewed, or we need to defend against a third person’s data perturbation. Ultimately, I aim to establish a framework to verify whether the model satisfies properties specified by users.